-
✔ R Markdown file: up-to-date
Great! Since the R Markdown file has been committed to the Git repository, you know the exact version of the code that produced these results.
-
✔ Environment: empty
Great job! The global environment was empty. Objects defined in the global environment can affect the analysis in your R Markdown file in unknown ways. For reproduciblity it’s best to always run the code in an empty environment.
-
✔ Seed: set.seed(20180618)
The command set.seed(20180618)
was run prior to running the code in the R Markdown file. Setting a seed ensures that any results that rely on randomness, e.g. subsampling or permutations, are reproducible.
-
✔ Session information: recorded
Great job! Recording the operating system, R version, and package versions is critical for reproducibility.
-
✔ Repository version: e84909d
Great! You are using Git for version control. Tracking code development and connecting the code version to the results is critical for reproducibility. The version displayed above was the version of the Git repository at the time these results were generated.
Note that you need to be careful to ensure that all relevant files for the analysis have been committed to Git prior to generating the results (you can use wflow_publish
or wflow_git_commit
). workflowr only checks the R Markdown file, but you know if there are other scripts or data files that it depends on. Below is the status of the Git repository when the results were generated:
Ignored files:
Ignored: .Rhistory
Ignored: .Rproj.user/
Ignored: R/.Rhistory
Ignored: analysis/.Rhistory
Ignored: analysis/pipeline/.Rhistory
Untracked files:
Untracked: ..gif
Untracked: .DS_Store
Untracked: R/.DS_Store
Untracked: analysis/.DS_Store
Untracked: analysis/bibliography.bib
Untracked: analysis/pipeline/large_sets.pdf
Untracked: analysis/pipeline/temp_ari.txt
Untracked: analysis/pipeline/temp_time.txt
Untracked: analysis/tutorial_cache/
Untracked: analysis/writeup/cite.bib
Untracked: analysis/writeup/cite.log
Untracked: analysis/writeup/paper.aux
Untracked: analysis/writeup/paper.bbl
Untracked: analysis/writeup/paper.blg
Untracked: analysis/writeup/paper.log
Untracked: analysis/writeup/paper.out
Untracked: analysis/writeup/paper.synctex.gz
Untracked: analysis/writeup/paper.tex
Untracked: analysis/writeup/writeup.aux
Untracked: analysis/writeup/writeup.bbl
Untracked: analysis/writeup/writeup.blg
Untracked: analysis/writeup/writeup.dvi
Untracked: analysis/writeup/writeup.log
Untracked: analysis/writeup/writeup.out
Untracked: analysis/writeup/writeup.synctex.gz
Untracked: analysis/writeup/writeup.tex
Untracked: analysis/writeup/writeup2.aux
Untracked: analysis/writeup/writeup2.bbl
Untracked: analysis/writeup/writeup2.blg
Untracked: analysis/writeup/writeup2.log
Untracked: analysis/writeup/writeup2.out
Untracked: analysis/writeup/writeup2.pdf
Untracked: analysis/writeup/writeup2.synctex.gz
Untracked: analysis/writeup/writeup2.tex
Untracked: analysis/writeup/writeup3.aux
Untracked: analysis/writeup/writeup3.log
Untracked: analysis/writeup/writeup3.out
Untracked: analysis/writeup/writeup3.synctex.gz
Untracked: analysis/writeup/writeup3.tex
Untracked: data/unnecessary_in_building/
Unstaged changes:
Modified: R/LSLSL.R
Modified: R/SLSL.R
Modified: R/SLSL_ref.R
Modified: R/tsne_spectral.R
Deleted: SCNoisyClustering_0.1.0.tar.gz
Deleted: analysis/correcting_detection_rate/Buettner.Rmd
Deleted: analysis/correcting_detection_rate/Buettner.pdf
Deleted: analysis/correcting_detection_rate/Pollen.R
Deleted: analysis/correcting_detection_rate/Pollen.Rmd
Deleted: analysis/correcting_detection_rate/Pollen.pdf
Deleted: analysis/correcting_detection_rate/Usoskin.Rmd
Deleted: analysis/correcting_detection_rate/Usoskin.pdf
Deleted: analysis/correcting_detection_rate/Usoskin_files/figure-latex/loess residuals-1.pdf
Deleted: analysis/correcting_detection_rate/Usoskin_files/figure-latex/pca_plot-1.pdf
Deleted: analysis/correcting_detection_rate/Usoskin_files/figure-latex/quantile_plot-1.pdf
Deleted: analysis/correcting_detection_rate/Usoskin_files/figure-latex/quantile_plot_log-1.pdf
Deleted: analysis/correcting_detection_rate/Usoskin_files/figure-latex/rg residuals-1.pdf
Deleted: analysis/correcting_detection_rate/Zeisel.R
Deleted: analysis/correcting_detection_rate/detectionrate_alldata.R
Deleted: analysis/correcting_detection_rate/detectionrate_pbmc.R
Deleted: analysis/correcting_detection_rate/sc.xls
Modified: analysis/pipeline/large_sets.Rmd
Modified: analysis/pipeline/pbmc_pipeline.R
Modified: analysis/pipeline/small_good_sets.Rmd
Modified: analysis/pipeline/small_good_sets_result.txt
Modified: analysis/pipeline/small_good_sets_time.txt
Modified: analysis/writeup/.DS_Store
Modified: src/SCNoisyClustering.cpp
Modified: src/SCNoisyClustering.o
Modified: src/SCNoisyClustering.so
Note that any generated files, e.g. HTML, png, CSS, etc., are not included in this status report because it is ok for generated content to have uncommitted changes.
Pollen
i = 2
load('../data/unnecessary_in_building/2_Pollen.RData')
X = as.matrix(Pollen$x)
truth = as.numeric(as.factor(Pollen$label))
numClust = length(unique(truth))
rm(Pollen)
logX = log(X+1)
det = colSums(X!=0) / nrow(X)
det2 = qr(cbind(rep(1,length(det)), det))
R = t(qr.resid(det2, t(logX)))
pca1 = irlba(R,2); pca2 = irlba(logX,2)
dat = data.frame(pc1=c(pca1$v[,1], pca2$v[,1]), detection.rate=rep(det, 2), label=rep(c("After correction", "Before correction"), each=nrow(pca1$v)), true.label=as.factor(rep(truth,2)))
ggplot(dat, aes(x=pc1, y=detection.rate, col=true.label)) + facet_grid(~label) + geom_point() + ggtitle("PCA")
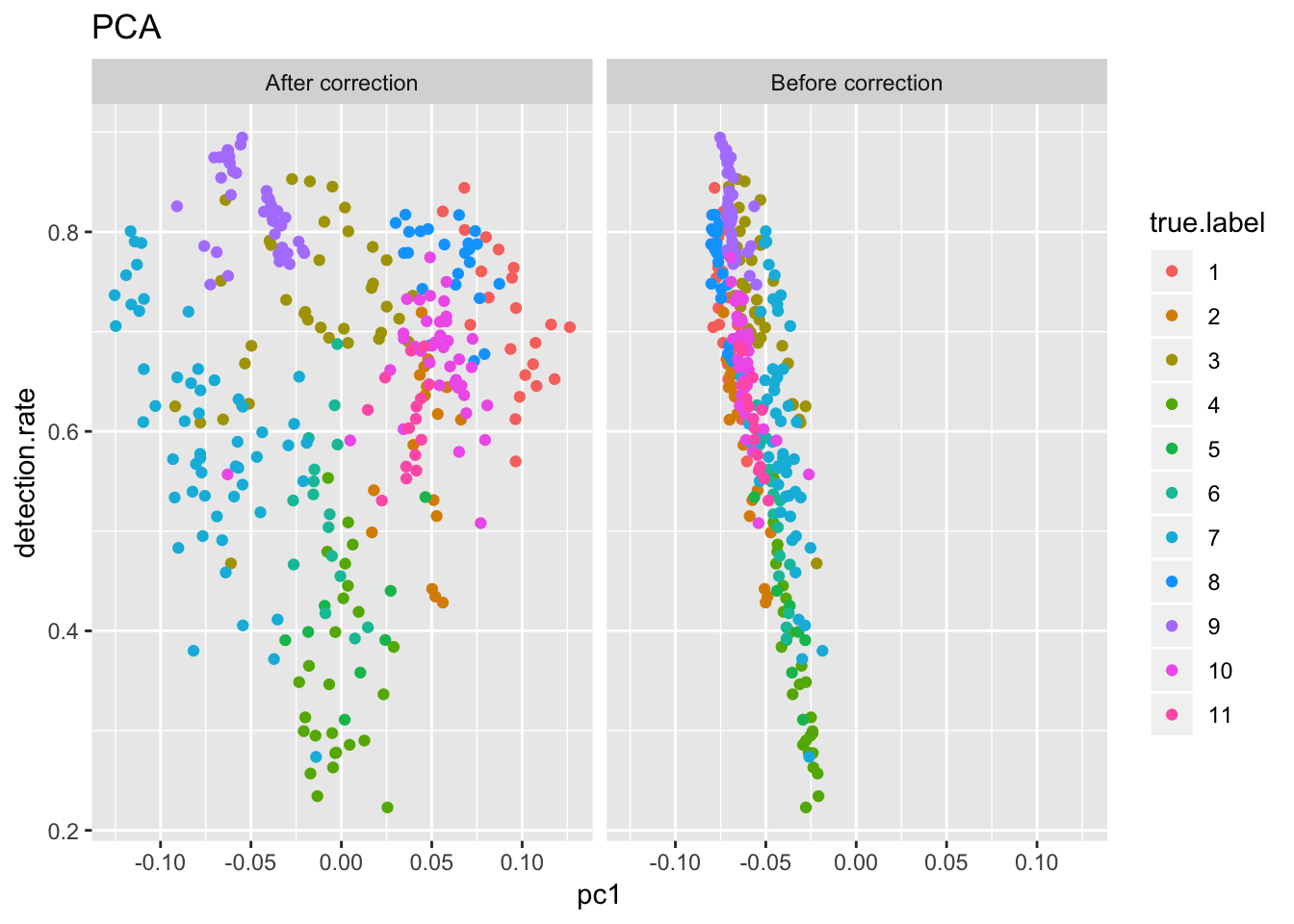
Expand here to see past versions of Pollen-1.png:
Version
|
Author
|
Date
|
b7e4475
|
tk382
|
2018-07-16
|
tsne1 = Rtsne(t(R))
tsne2 = Rtsne(t(logX))
dat = data.frame(v1 = c(tsne1$Y[,1], tsne2$Y[,1]), v2 = c(tsne1$Y[,2], tsne2$Y[,2]), label=rep(c("After correction", "Before correction"), each=nrow(tsne1$Y)), true.label = as.factor(rep(truth, 2)))
ggplot(dat, aes(x=v1, y=v2, col=true.label)) + facet_grid(~label) + geom_point() + ggtitle("tSNE")
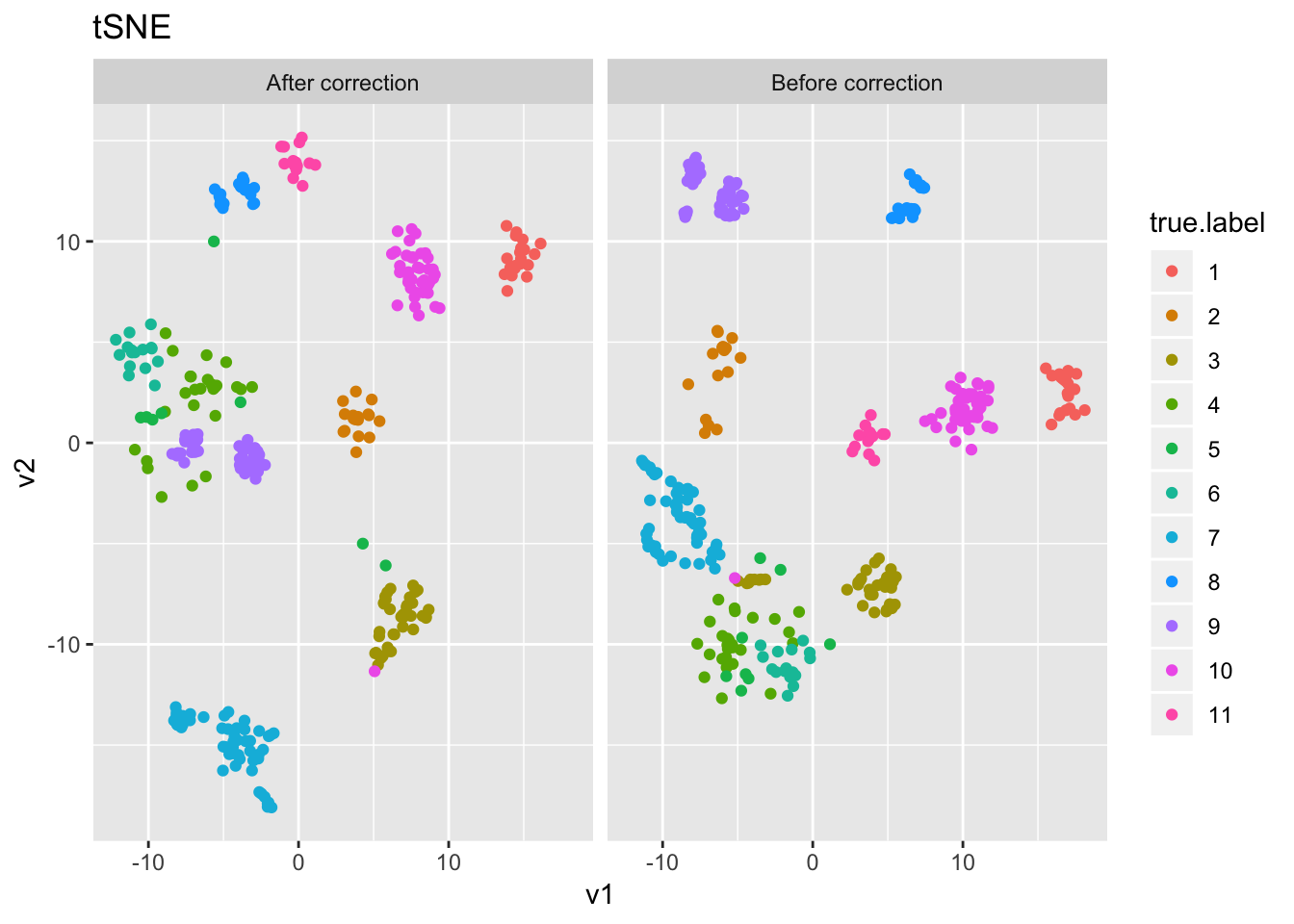
Expand here to see past versions of Pollen-2.png:
Version
|
Author
|
Date
|
b7e4475
|
tk382
|
2018-07-16
|
set.seed(1)
res1 = SLSL(X, log=T, filter=F, correct_detection_rate = T, numClust = numClust)
adj.rand.index(res1$result, truth)
[1] 0.7931186
set.seed(1)
res2 = SLSL(X, log=T, filter=F, correct_detection_rate = F, numClust = numClust)
adj.rand.index(res2$result, truth)
[1] 0.8177593
Usoskin
i = 3
load('../data/unnecessary_in_building/3_Usoskin.RData')
X = as.matrix(Usoskin$X)
truth = as.numeric(as.factor(as.character(Usoskin$lab1)))
numClust = 4
rm(Usoskin)
logX = log(X+1)
det = colSums(X!=0) / nrow(X)
plot(irlba(logX,1)$v[,1]~log(det))
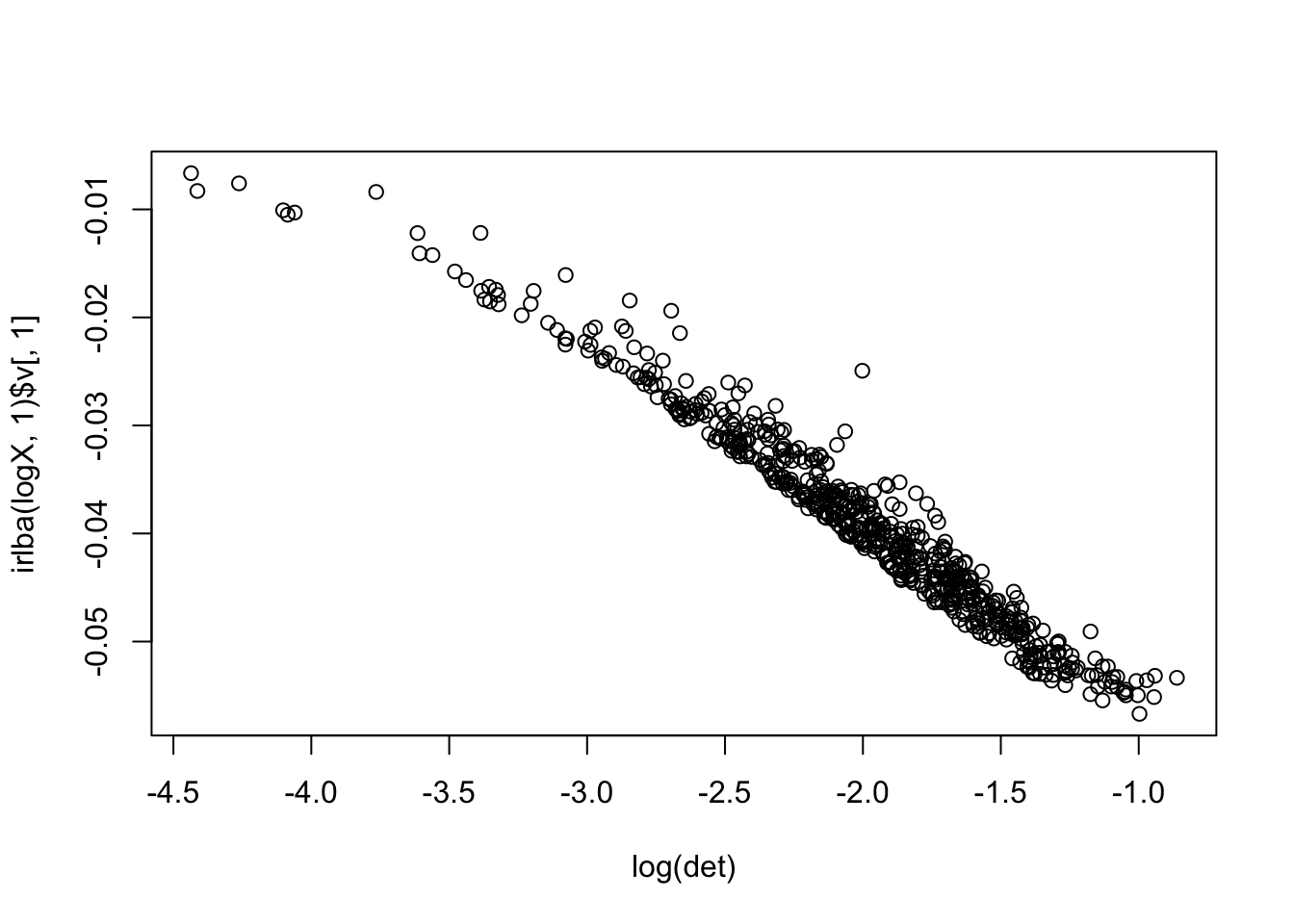
Expand here to see past versions of Usoskin-1.png:
Version
|
Author
|
Date
|
b7e4475
|
tk382
|
2018-07-16
|
det2 = qr(cbind(rep(1, length(det)), log(det)))
R = t(qr.resid(det2, t(logX)))
pca1 = irlba(R,2); pca2 = irlba(logX,2)
dat = data.frame(pc1=c(pca1$v[,1], pca2$v[,1]), detection.rate=rep(det, 2), label=rep(c("After correction", "Before correction"), each=nrow(pca1$v)), true.label=as.factor(rep(truth,2)))
ggplot(dat, aes(x=pc1, y=detection.rate, col=true.label)) + facet_grid(~label) + geom_point() + ggtitle("PCA")
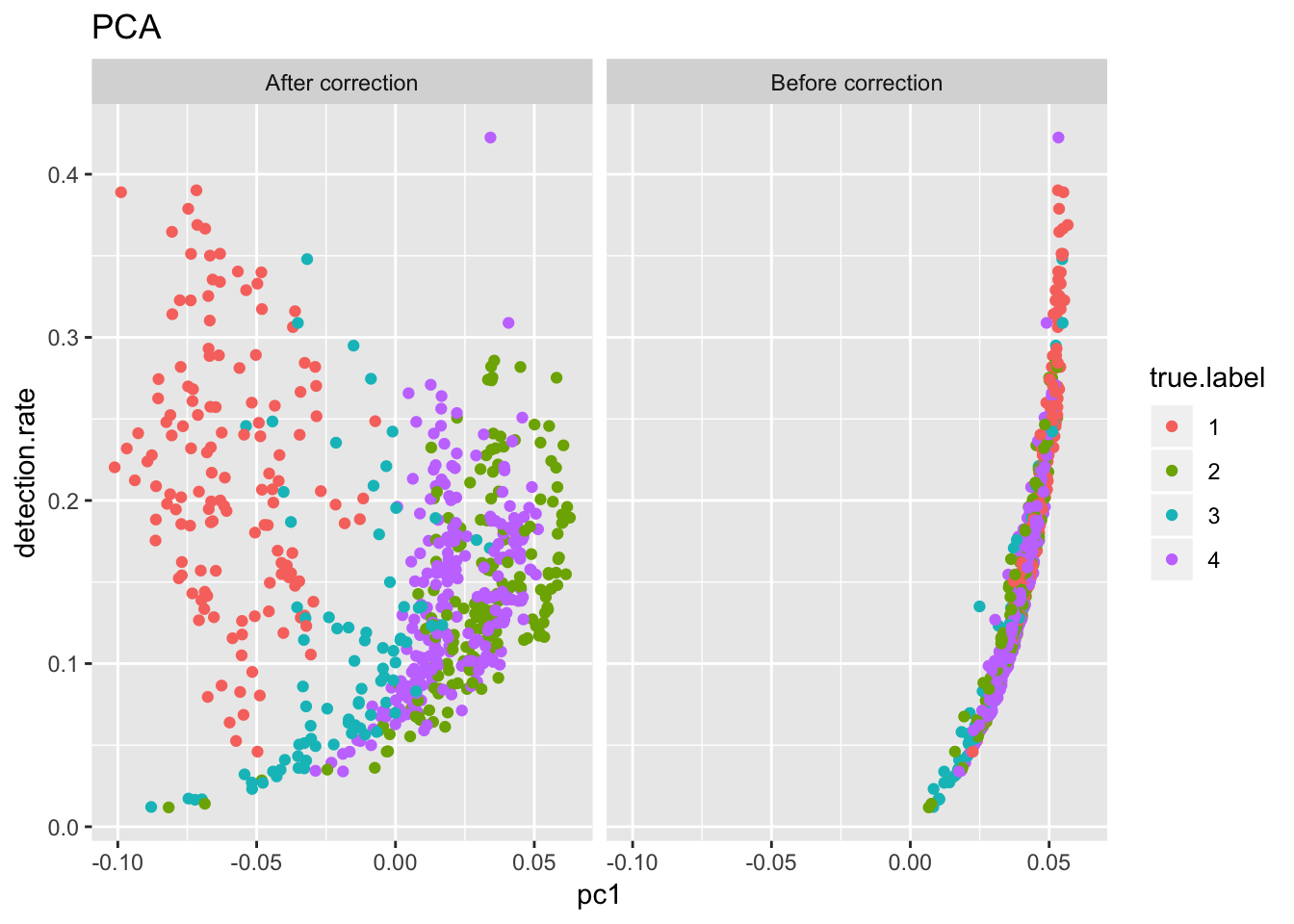
Expand here to see past versions of Usoskin-2.png:
Version
|
Author
|
Date
|
b7e4475
|
tk382
|
2018-07-16
|
tsne1 = Rtsne(t(R), perplexity=20)
tsne2 = Rtsne(t(logX))
dat = data.frame(v1 = c(tsne1$Y[,1], tsne2$Y[,1]), v2 = c(tsne1$Y[,2], tsne2$Y[,2]), label=rep(c("After correction", "Before correction"), each=nrow(tsne1$Y)), true.label = as.factor(rep(truth, 2)))
ggplot(dat, aes(x=v1, y=v2, col=true.label)) + facet_grid(~label) + geom_point() + ggtitle("tSNE")
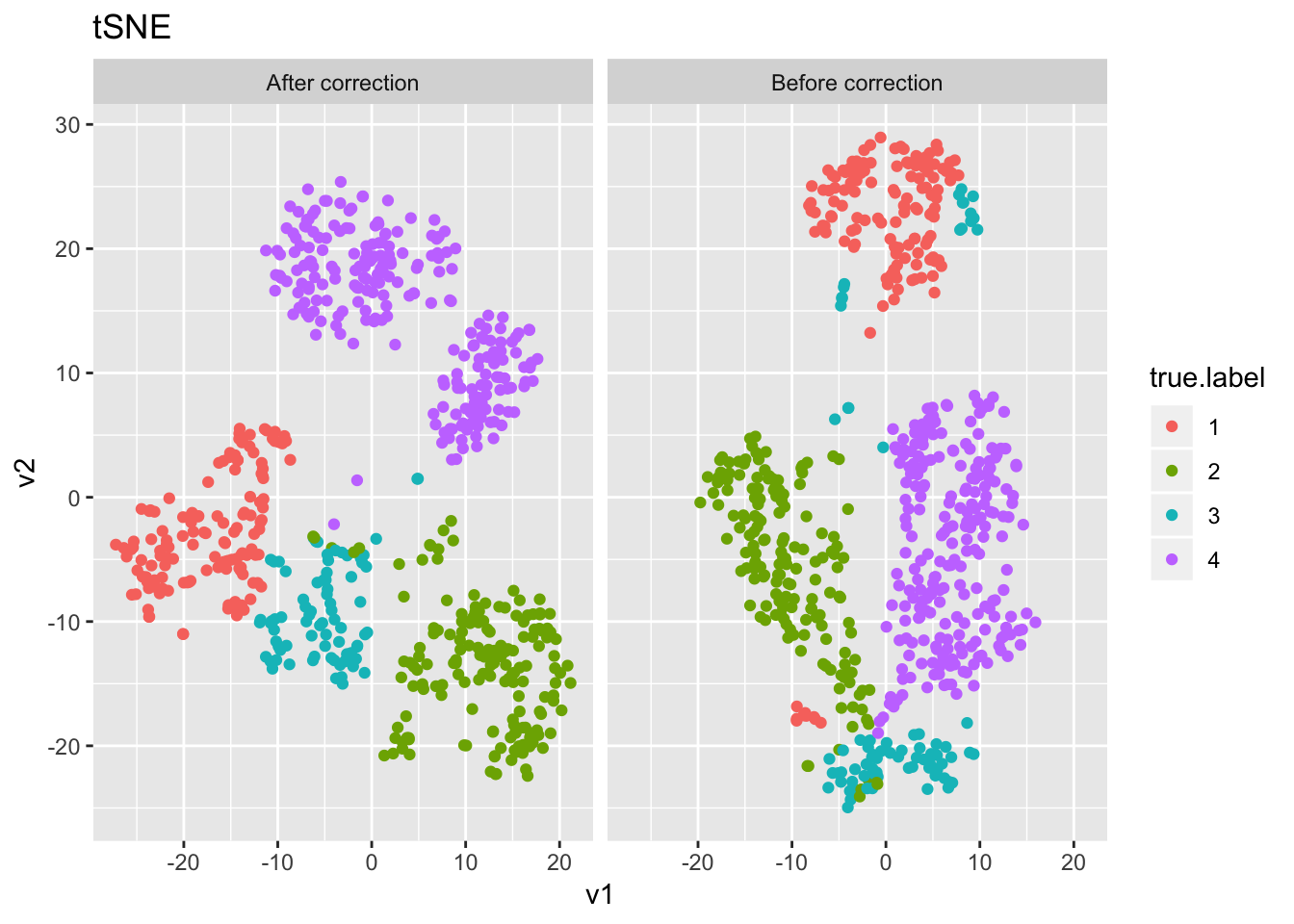
Expand here to see past versions of Usoskin-3.png:
Version
|
Author
|
Date
|
b7e4475
|
tk382
|
2018-07-16
|
set.seed(1)
res1 = SLSL(X, log=T, filter=F, correct_detection_rate = T, numClust = numClust)
adj.rand.index(res1$result, truth)
[1] 0.7947954
set.seed(1)
res2 = SLSL(X, log=T, filter=F, correct_detection_rate = F, numClust = numClust)
adj.rand.index(res2$result, truth)
[1] 0.6218291
Buettner
i = 4
#read data
load('../data/unnecessary_in_building/4_Buettner.RData')
X = as.matrix(Buettner$X)
truth = as.numeric(as.factor(Buettner$label))
numClust = 3
rm(Buettner)
logX = log(X+1)
det = colSums(X!=0) / nrow(X)
det2 = qr(det)
R = t(qr.resid(det2, t(logX)))
pca1 = irlba(R,2); pca2 = irlba(logX,2)
dat = data.frame(pc1=c(pca1$v[,1], pca2$v[,1]), detection.rate=rep(det, 2), label=rep(c("After correction", "Before correction"), each=nrow(pca1$v)), true.label=as.factor(rep(truth,2)))
ggplot(dat, aes(x=pc1, y=detection.rate, col=true.label)) + facet_grid(~label) + geom_point() + ggtitle("PCA")
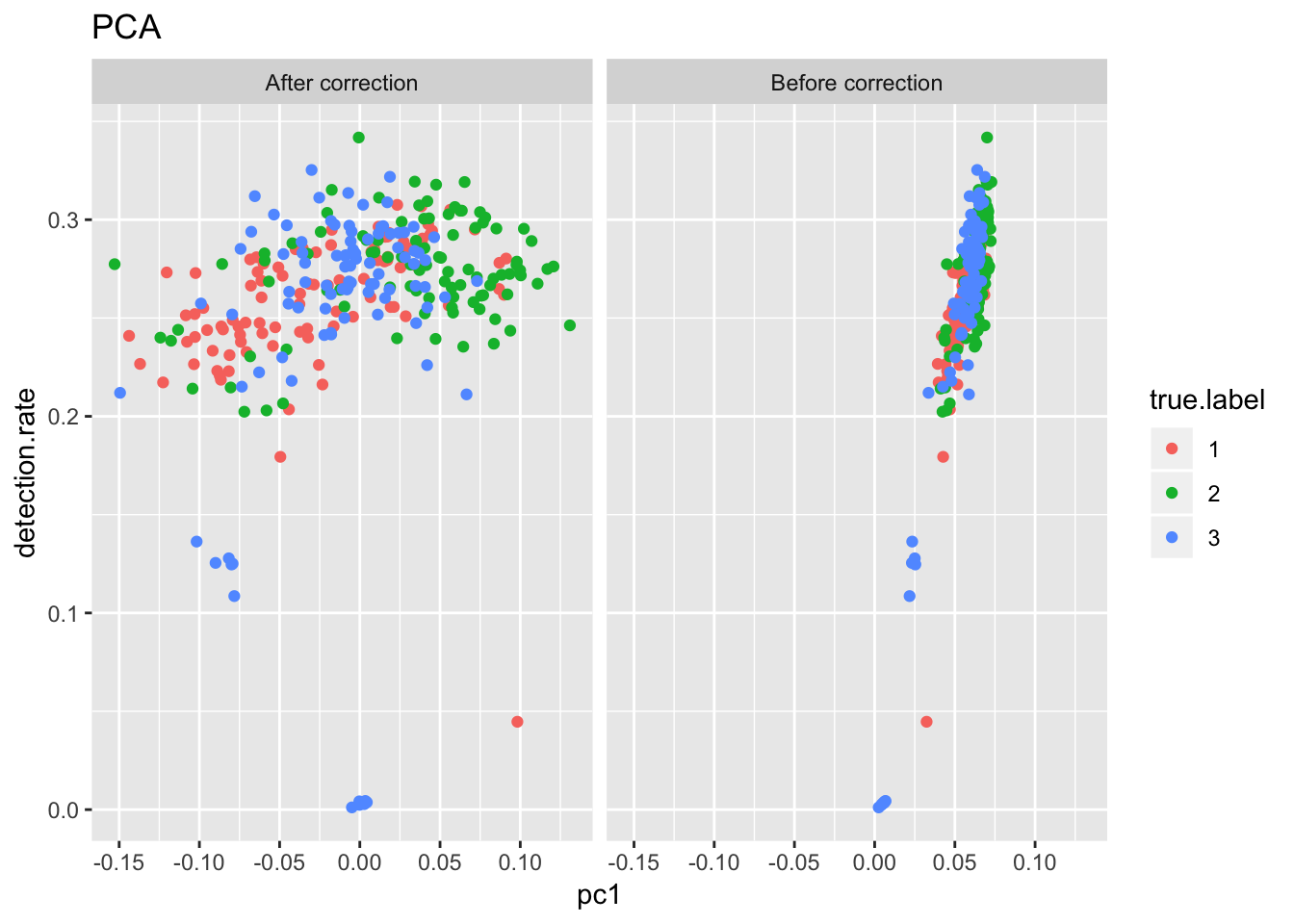
Expand here to see past versions of Buettner-1.png:
Version
|
Author
|
Date
|
b7e4475
|
tk382
|
2018-07-16
|
tsne1 = Rtsne(t(R), perplexity=20)
tsne2 = Rtsne(t(logX), perplexity=20)
dat = data.frame(v1 = c(tsne1$Y[,1], tsne2$Y[,1]), v2 = c(tsne1$Y[,2], tsne2$Y[,2]), label=rep(c("After correction", "Before correction"), each=nrow(tsne1$Y)), true.label = as.factor(rep(truth, 2)))
ggplot(dat, aes(x=v1, y=v2, col=true.label)) + facet_grid(~label) + geom_point() + ggtitle("tSNE")
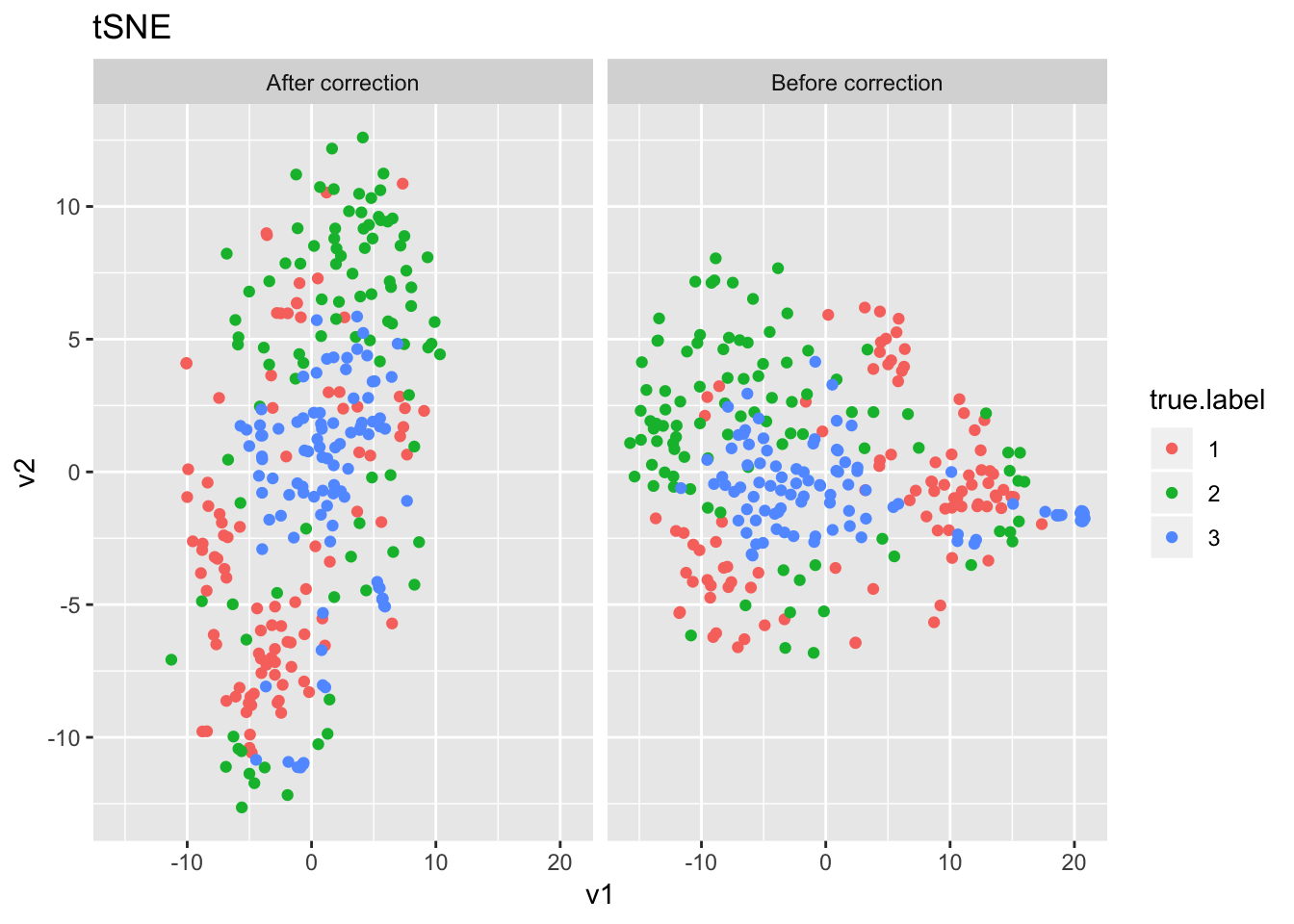
Expand here to see past versions of Buettner-2.png:
Version
|
Author
|
Date
|
b7e4475
|
tk382
|
2018-07-16
|
set.seed(1)
res1 = SLSL(X, log=T, filter=F, correct_detection_rate = T, numClust = numClust)
adj.rand.index(res1$result, truth)
[1] 0.3806902
set.seed(1)
res2 = SLSL(X, log=T, filter=F, correct_detection_rate = F, numClust = numClust)
adj.rand.index(res2$result, truth)
[1] 0.3859772
Yan
i = 5
load('../data/unnecessary_in_building/5_Yan.rda')
X = as.matrix(yan)
truth = as.character(ann$cell_type1)
truth = as.numeric(as.factor(truth))
numClust = 6
rm(ann, yan)
logX = log(X+1)
det = colSums(X!=0) / nrow(X)
det2 = qr(det)
R = t(qr.resid(det2, t(logX)))
pca1 = irlba(R,2); pca2 = irlba(logX,2)
dat = data.frame(pc1=c(pca1$v[,1], pca2$v[,1]), detection.rate=rep(det, 2), label=rep(c("After correction", "Before correction"), each=nrow(pca1$v)), true.label=as.factor(rep(truth,2)))
ggplot(dat, aes(x=pc1, y=detection.rate, col=true.label)) + facet_grid(~label) + geom_point() + ggtitle("PCA")
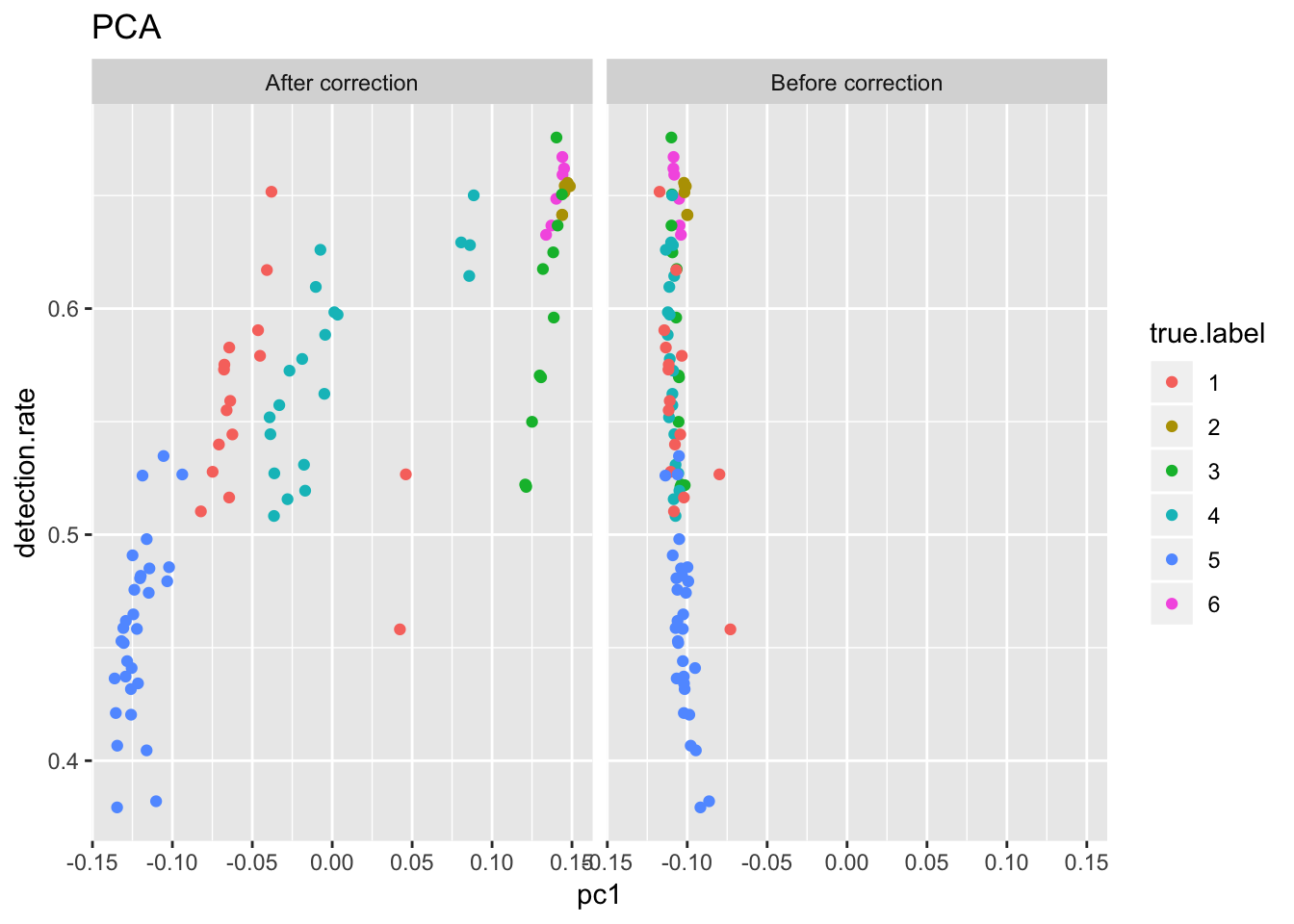
Expand here to see past versions of Yan-1.png:
Version
|
Author
|
Date
|
b7e4475
|
tk382
|
2018-07-16
|
tsne1 = Rtsne(t(R), perplexity=20)
tsne2 = Rtsne(t(logX), perplexity=20)
dat = data.frame(v1 = c(tsne1$Y[,1], tsne2$Y[,1]), v2 = c(tsne1$Y[,2], tsne2$Y[,2]), label=rep(c("After correction", "Before correction"), each=nrow(tsne1$Y)), true.label = as.factor(rep(truth, 2)))
ggplot(dat, aes(x=v1, y=v2, col=true.label)) + facet_grid(~label) + geom_point() + ggtitle("tSNE")
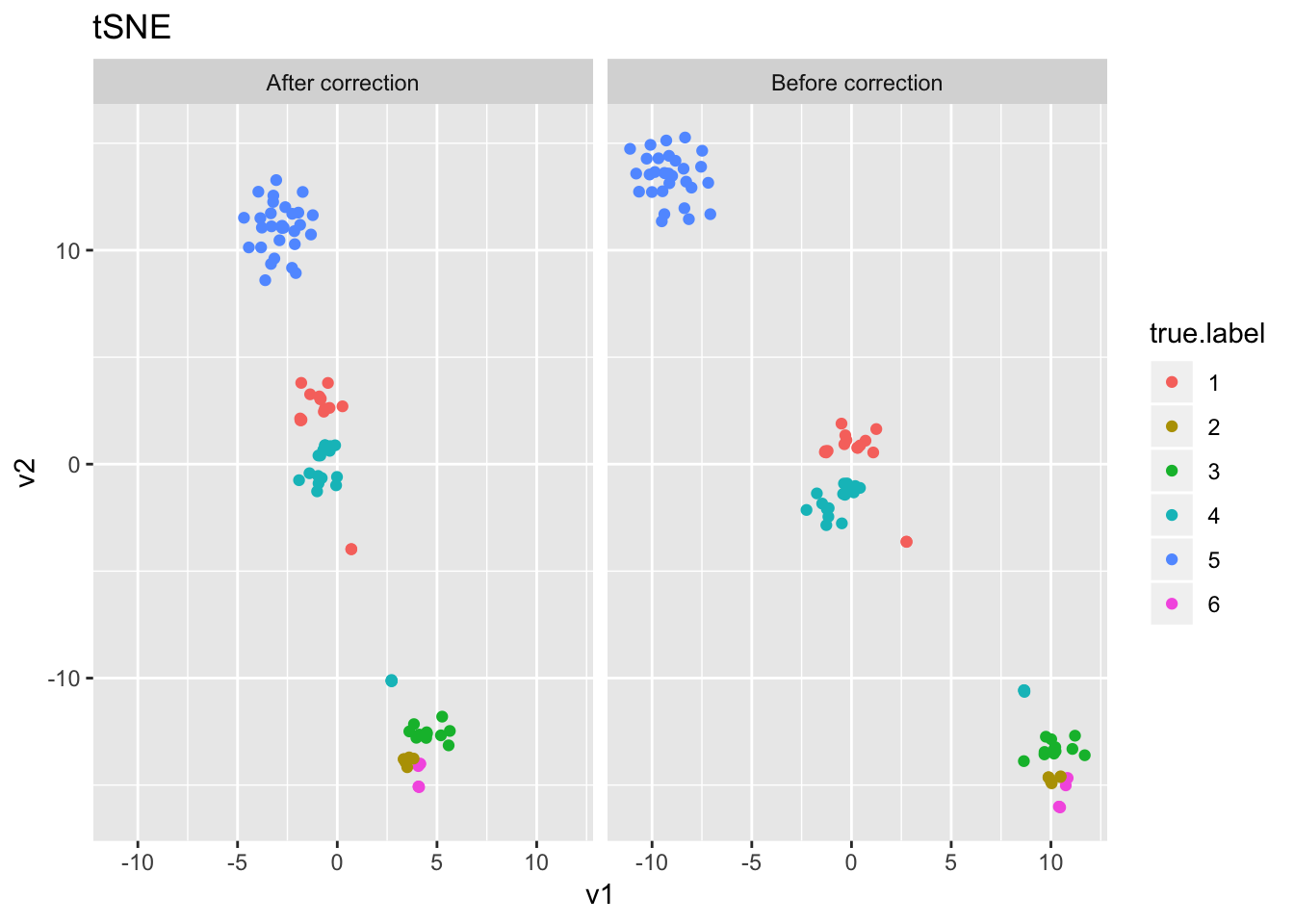
Expand here to see past versions of Yan-2.png:
set.seed(1)
res1 = SLSL(X, log=T, filter=F, correct_detection_rate = T, numClust = numClust)
adj.rand.index(res1$result, truth)
[1] 0.8954618
set.seed(1)
res2 = SLSL(X, log=T, filter=F, correct_detection_rate = F, numClust = numClust)
adj.rand.index(res2$result, truth)
[1] 0.5991576
Treutlein
i = 6
load('../data/unnecessary_in_building/6_Treutlein.rda')
X = as.matrix(treutlein)
truth = as.numeric(colnames(treutlein))
ind = sort(truth, index.return=TRUE)$ix
X = X[,ind]
truth = truth[ind]
numClust = length(unique(truth))
rm(treutlein)
logX = log(X+1)
det = colSums(X!=0) / nrow(X)
det2 = qr(cbind(log(det), rep(1, length(det))))
R = t(qr.resid(det2, t(logX)))
pca1 = irlba(R,2); pca2 = irlba(logX,2)
dat = data.frame(pc1=c(pca1$v[,1], pca2$v[,1]), detection.rate=rep(det, 2), label=rep(c("After correction", "Before correction"), each=nrow(pca1$v)), true.label=as.factor(rep(truth,2)))
ggplot(dat, aes(x=pc1, y=detection.rate, col=true.label)) + facet_grid(~label) + geom_point() + ggtitle("PCA")
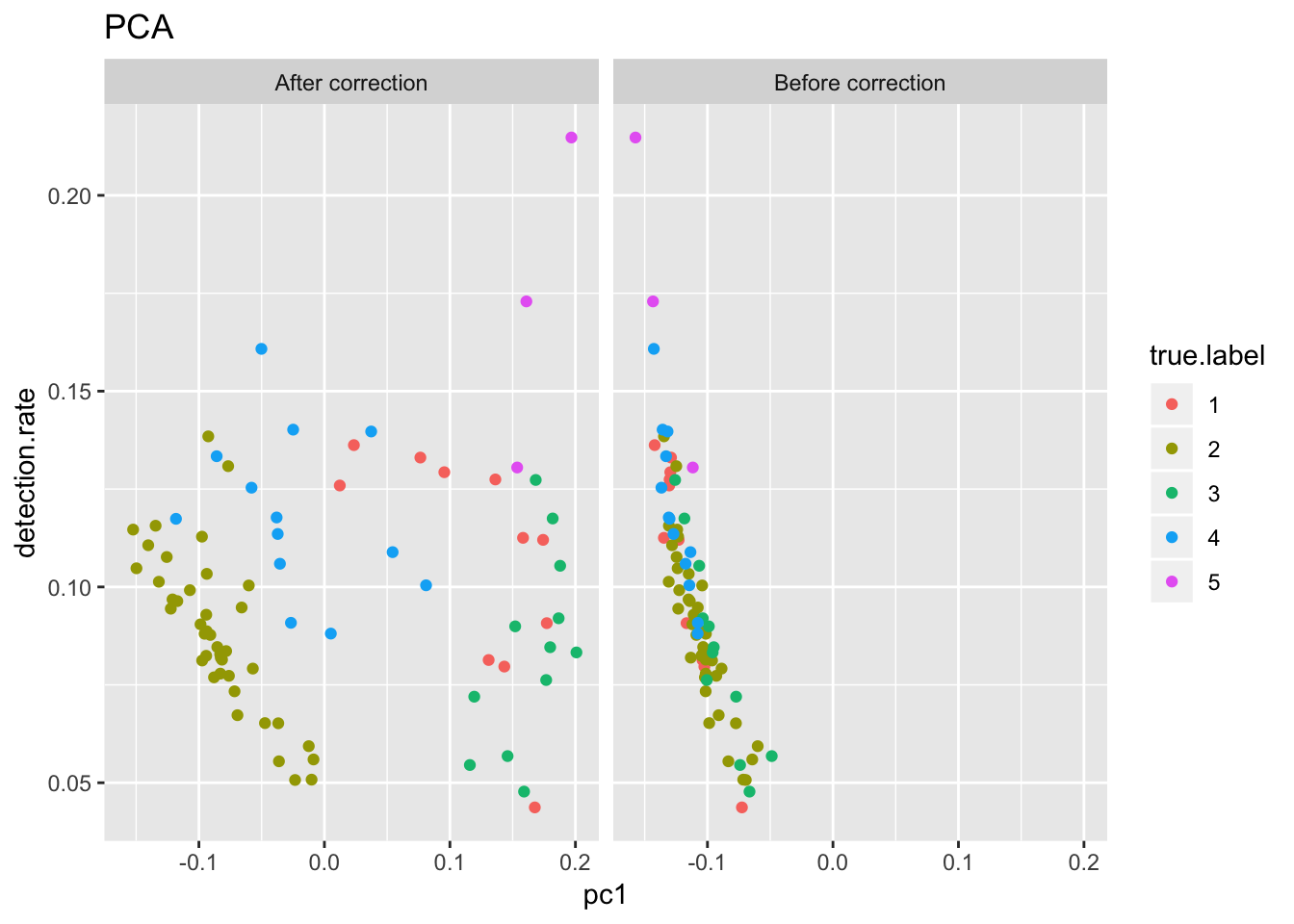
Expand here to see past versions of Treutlein-1.png:
tsne1 = Rtsne(t(R), perplexity=10)
tsne2 = Rtsne(t(logX), perplexity=10)
dat = data.frame(v1 = c(tsne1$Y[,1], tsne2$Y[,1]), v2 = c(tsne1$Y[,2], tsne2$Y[,2]), label=rep(c("After correction", "Before correction"), each=nrow(tsne1$Y)), true.label = as.factor(rep(truth, 2)))
ggplot(dat, aes(x=v1, y=v2, col=true.label)) + facet_grid(~label) + geom_point() + ggtitle("tSNE")
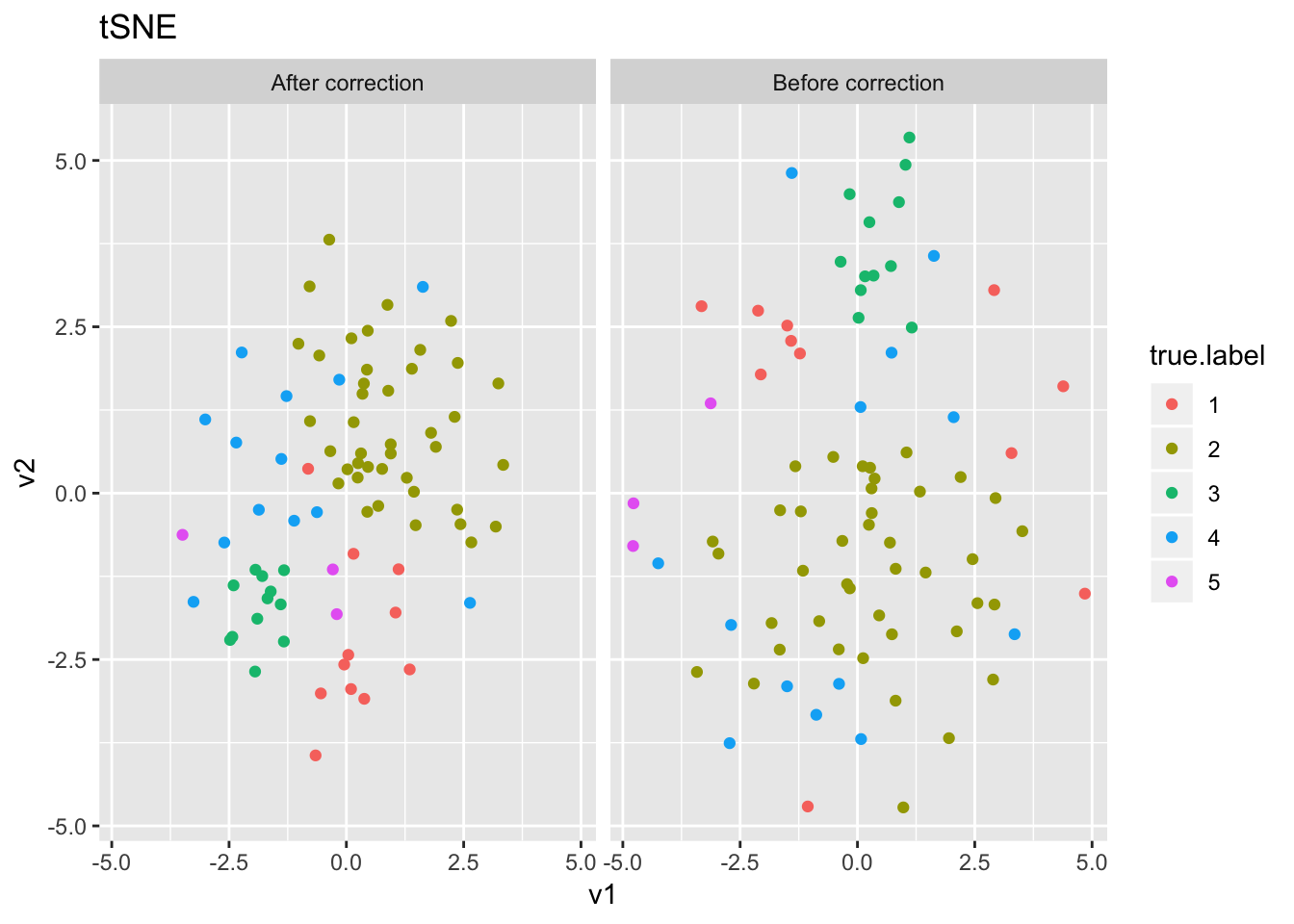
Expand here to see past versions of Treutlein-2.png:
set.seed(1)
res1 = SLSL(X, log=T, filter=F, correct_detection_rate = T, numClust = numClust)
adj.rand.index(res1$result, truth)
[1] 0.3490127
set.seed(1)
res2 = SLSL(X, log=T, filter=F, correct_detection_rate = F, numClust = numClust)
adj.rand.index(res2$result, truth)
[1] 0.4146639
Chu (cell type)
i = 7
load('../data/unnecessary_in_building/7_Chu_celltype.Rdata')
X = as.matrix(Chu_celltype$X)
truth = as.numeric(as.factor(Chu_celltype$label))
numClust = 7
rm(Chu_celltype)
logX = log(X+1)
det = colSums(X!=0) / nrow(X)
det2 = qr(det)
R = t(qr.resid(det2, t(logX)))
pca1 = irlba(R,2); pca2 = irlba(logX,2)
dat = data.frame(pc1=c(pca1$v[,1], pca2$v[,1]), detection.rate=rep(det, 2), label=rep(c("After correction", "Before correction"), each=nrow(pca1$v)), true.label=as.factor(rep(truth,2)))
ggplot(dat, aes(x=pc1, y=detection.rate, col=true.label)) + facet_grid(~label) + geom_point() + ggtitle("PCA")
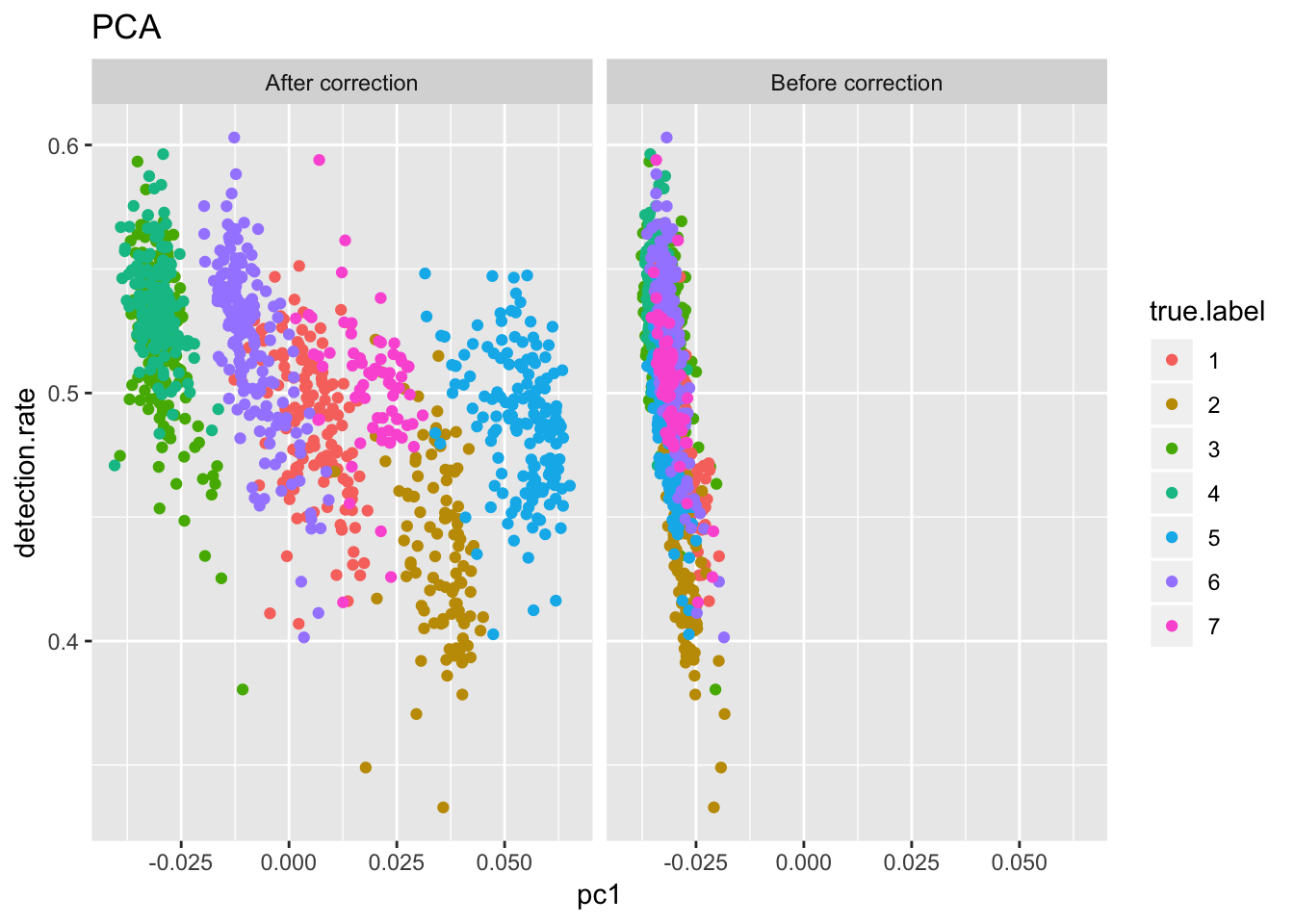
Expand here to see past versions of Chu_celltype-1.png:
Version
|
Author
|
Date
|
b7e4475
|
tk382
|
2018-07-16
|
tsne1 = Rtsne(t(R))
tsne2 = Rtsne(t(logX))
dat = data.frame(v1 = c(tsne1$Y[,1], tsne2$Y[,1]), v2 = c(tsne1$Y[,2], tsne2$Y[,2]), label=rep(c("After correction", "Before correction"), each=nrow(tsne1$Y)), true.label = as.factor(rep(truth, 2)))
ggplot(dat, aes(x=v1, y=v2, col=true.label)) + facet_grid(~label) + geom_point() + ggtitle("tSNE")
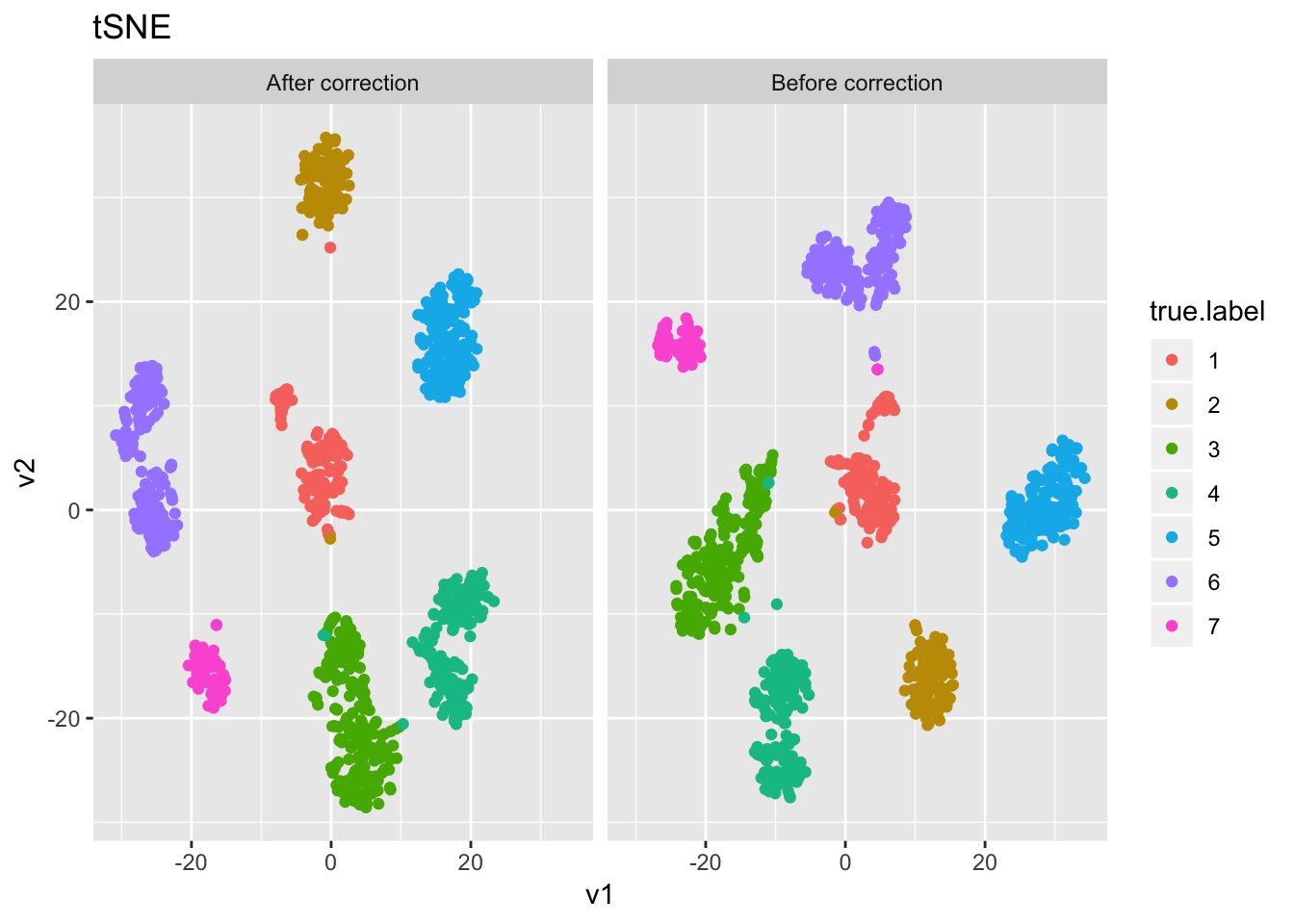
Expand here to see past versions of Chu_celltype-2.png:
set.seed(1)
res1 = SLSL(X, log=T, filter=F, correct_detection_rate = T, numClust = numClust)
adj.rand.index(res1$result, truth)
[1] 0.798453
set.seed(1)
res2 = SLSL(X, log=T, filter=F, correct_detection_rate = F, numClust = numClust)
adj.rand.index(res2$result, truth)
[1] 0.9964407
Chu (timecourse)
i = 8
load('../data/unnecessary_in_building/8_Chu_timecourse.Rdata')
X = as.matrix(Chu_timecourse$X)
truth = as.numeric(as.factor(Chu_timecourse$label))
numClust = length(unique(truth))
logX = log(X+1)
det = colSums(X!=0) / nrow(X)
det2 = qr(det)
R = t(qr.resid(det2, t(logX)))
pca1 = irlba(R,2); pca2 = irlba(logX,2)
dat = data.frame(pc1=c(pca1$v[,1], pca2$v[,1]), detection.rate=rep(det, 2), label=rep(c("After correction", "Before correction"), each=nrow(pca1$v)), true.label=as.factor(rep(truth,2)))
ggplot(dat, aes(x=pc1, y=detection.rate, col=true.label)) + facet_grid(~label) + geom_point() + ggtitle("PCA")
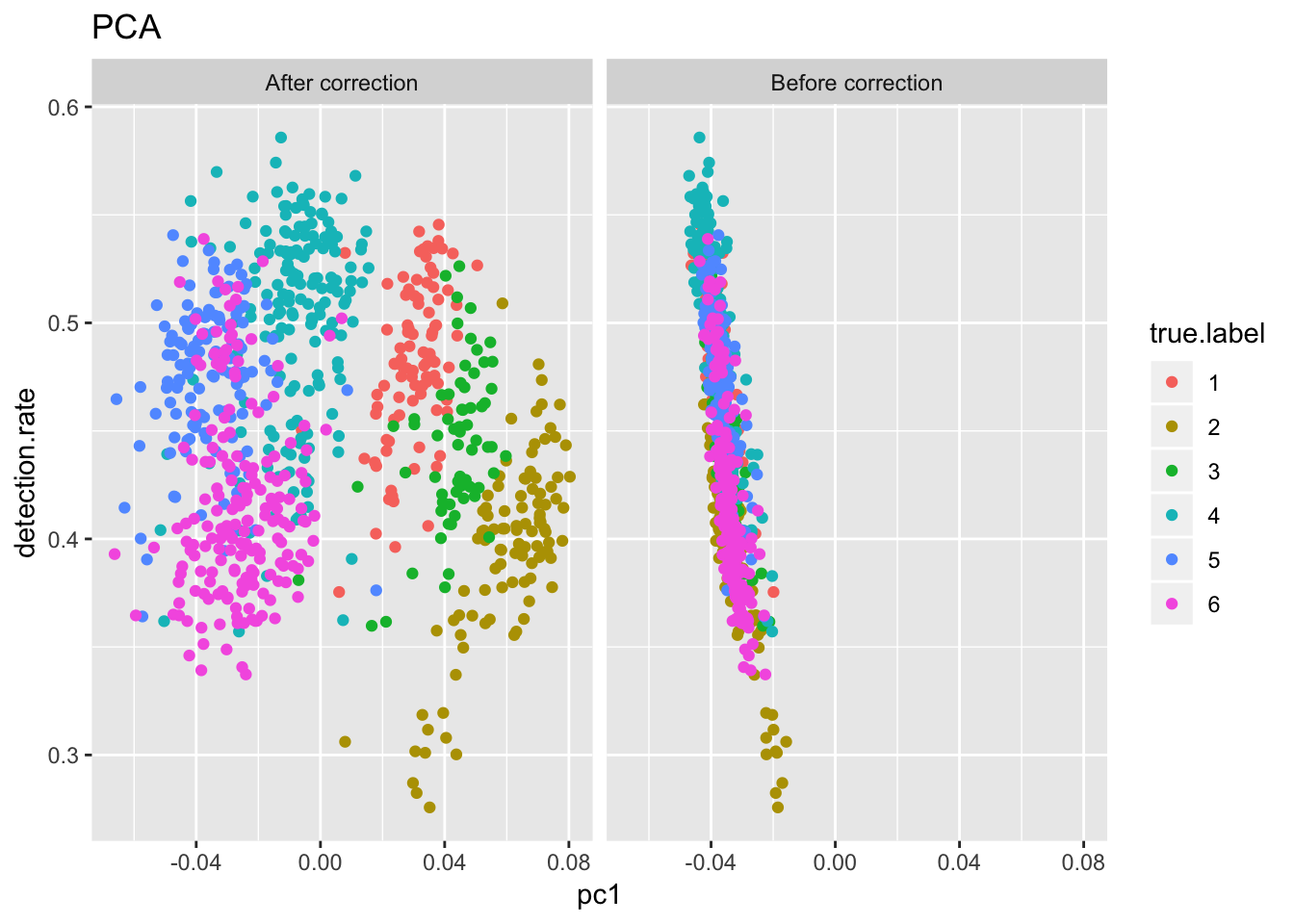
Expand here to see past versions of Chu_timecourse-1.png:
tsne1 = Rtsne(t(R))
tsne2 = Rtsne(t(logX))
dat = data.frame(v1 = c(tsne1$Y[,1], tsne2$Y[,1]), v2 = c(tsne1$Y[,2], tsne2$Y[,2]), label=rep(c("After correction", "Before correction"), each=nrow(tsne1$Y)), true.label = as.factor(rep(truth, 2)))
ggplot(dat, aes(x=v1, y=v2, col=true.label)) + facet_grid(~label) + geom_point() + ggtitle("tSNE")
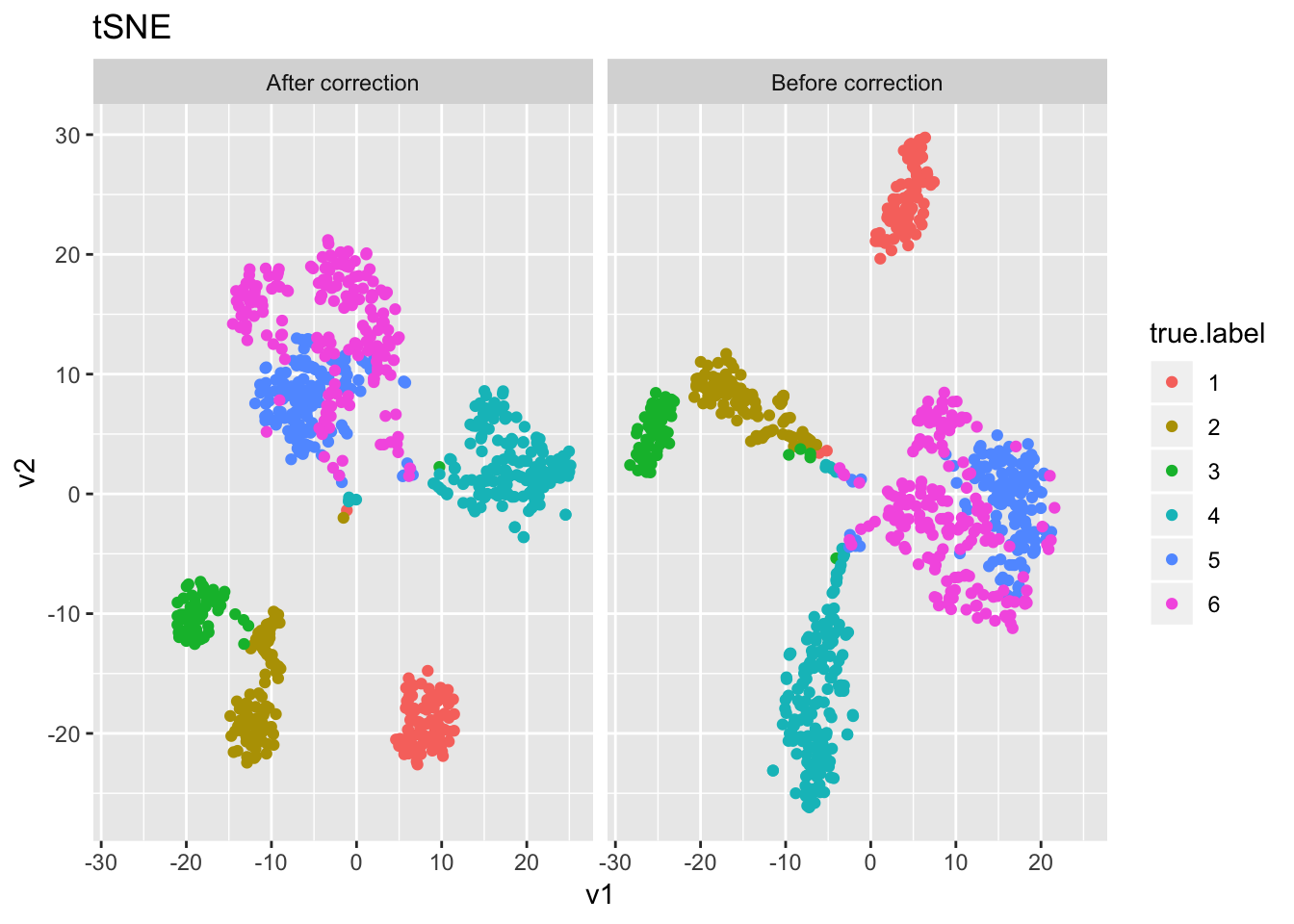
Expand here to see past versions of Chu_timecourse-2.png:
set.seed(1)
res1 = SLSL(X, log=T, filter=F, correct_detection_rate = T, numClust = numClust)
adj.rand.index(res1$result, truth)
[1] 0.7241204
set.seed(1)
res2 = SLSL(X, log=T, filter=F, correct_detection_rate = F, numClust = numClust)
adj.rand.index(res2$result, truth)
[1] 0.6174572